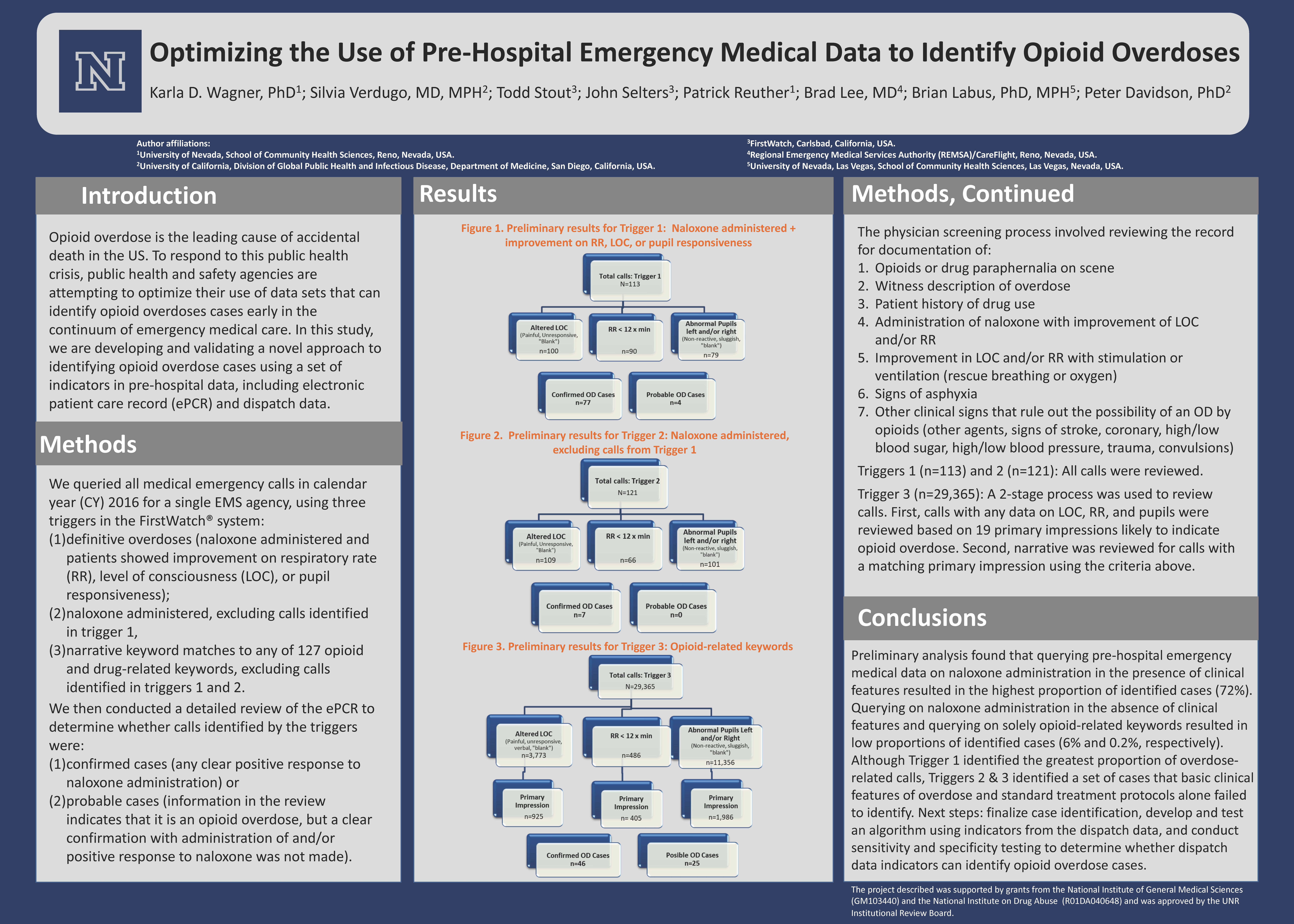
Abstract
Objectives: Opioid overdose is the leading cause of accidental death in the US. To respond to this public health crisis, public health and safety agencies are attempting to optimize their use of data sets that can identify opioid overdoses cases early in the continuum of emergency medical care. In this study, we are developing and validating a novel approach to identifying opioid overdose cases using a set of indicators from pre-hospital data, including the electronic patient care record (ePCR), call taking, and dispatch data.
Methods: We queried all medical emergency calls in calendar year (CY) 2016 for a single EMS agency, using three triggers in the FirstWatch system: (1) definitive overdoses (naloxone administered and patients showed improvement on respiratory rate (RR), level of consciousness (LOC), or pupil responsiveness); (2) naloxone administered, excluding calls identified in trigger 1, (3) narrative keyword matches to any of 127 opioid and drug-related keywords, excluding calls identified in triggers 1 and 2. We then conducted a detailed review of the electronic patient care record (ePCR) to determine whether calls identified by the triggers were likely to be opioid overdoses, and applied two criteria: (1) confirmed case (any clear positive response to naloxone administration and clinical features of opioid overdose) or (2) probable case (information in the review indicates that it is an opioid overdose, but a clear confirmation with administration of and/or positive response to naloxone was not made).
Results: Preliminary results suggest that the triggers identified a total of 29,599 calls for CY 2016; trigger 1 yielded 113, trigger 2 yielded 121, and trigger 3 yielded 29,365 calls. Upon review, trigger 1 yielded 77/113 confirmed and 4/113 probable overdoses; trigger 2 yielded 7/121 confirmed and 0/121 probable overdoses; and trigger 3 yielded 46/29,365 confirmed and 25/29,365 probable overdoses.
Conclusions: Preliminary analysis found that querying pre-hospital emergency medical data on naloxone administration in the presence of clinical features (improvement in LOC, RR, or pupil responsiveness) resulted in the highest proportion of identified cases (72%). Querying on naloxone administration in the absence of clinical features and querying on solely opioid-related keywords resulted in low proportions of identified cases (6% and 0.2%, respectively). Although Trigger 1 identified the greatest proportion of overdose-related calls, Triggers 2 & 3 identified a set of cases that basic clinical features of overdose and standard treatment protocols alone failed to identify. Next steps: we will refine the triggers to finalize case identification, develop and test an algorithm that includes indicators from the call taking and dispatch data, and conduct sensitivity and specificity testing to determine whether call taking and dispatch data indicators can identify opioid overdose cases.